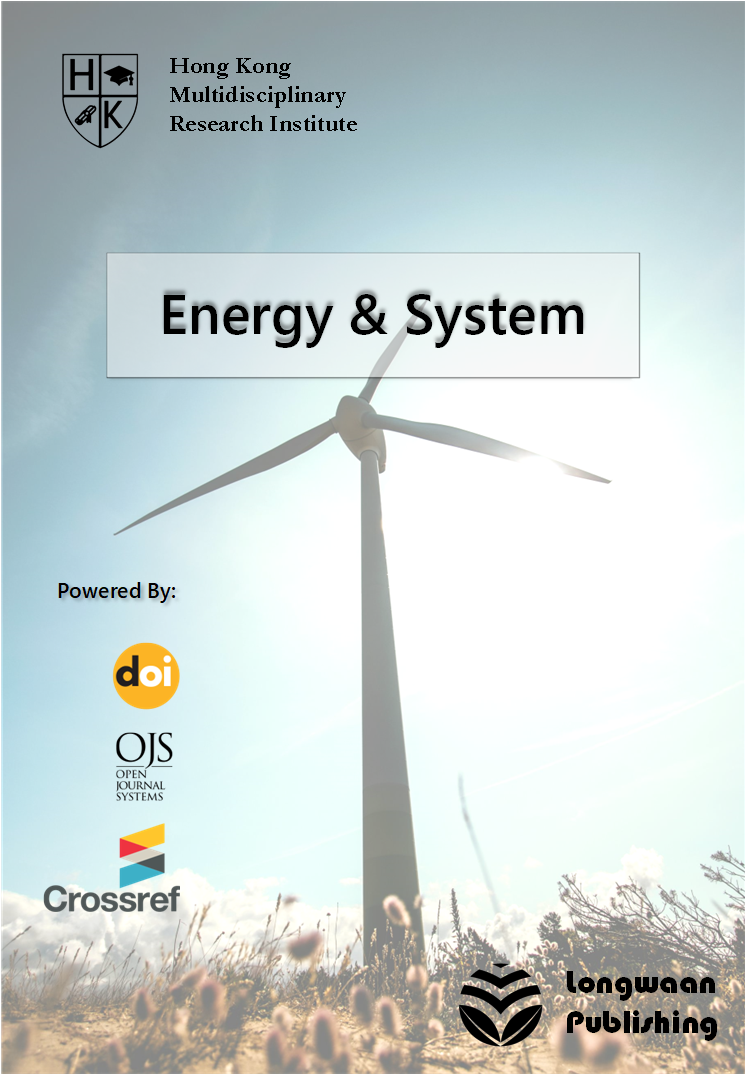
Published 2024-12-10
Keywords
- Electrical Vehicles,
- Battery,
- Supply Chain,
- Optimization,
- Machine Learning
How to Cite
Abstract
This paper presents a dynamic optimization model for the electric vehicle (EV) battery supply chain, addressing the critical need for efficient logistics amidst fluctuating demand and market conditions. The growing adoption of EVs necessitates robust supply chain mechanisms to ensure timely and cost-effective battery delivery. This task is challenging due to the complexity of real-time adjustments required in logistics, considering factors such as transportation delays, battery availability, and cost constraints. We develop a model that dynamically adjusts supply chain logistics in real-time, leveraging advanced optimization techniques including reinforcement learning, stochastic programming, and robust optimization. We validate our approach through extensive experiments under various demand scenarios, demonstrating significant improvements in supply chain efficiency, cost savings, and battery delivery speed. Our results highlight the effectiveness of our dynamic optimization approach in managing the complexities of the EV battery supply chain.
References
- G. Byeon, T. Yoon, S. Oh, and G. Jang, ‘Energy management strategy of the DC distribution system in buildings using the EV service model’, IEEE Transactions on Power Electronics, vol. 28, no. 4, pp. 1544–1554, 2012.
- T. Boström, B. Babar, J. B. Hansen, and C. Good, ‘The pure PV-EV energy system–A conceptual study of a nationwide energy system based solely on photovoltaics and electric vehicles’, Smart Energy, vol. 1, p. 100001, 2021.
- M. Centeno-Telleria, H. Yue, J. Carrol, J. I. Aizpurua, and M. Penalba, ‘O&M-aware techno-economic assessment for floating offshore wind farms: A geospatial evaluation off the North Sea and the Iberian Peninsula’, Applied Energy, vol. 371, p. 123684, Oct. 2024, doi: 10.1016/j.apenergy.2024.123684.
- E. Doroudchi, K. Alanne, Ö. Okur, J. Kyyrä, and M. Lehtonen, ‘Approaching net zero energy housing through integrated EV’, Sustainable Cities and Society, vol. 38, pp. 534–542, 2018.
- E. Cheynet, L. Li, and Z. Jiang, ‘Metocean conditions at two Norwegian sites for development of offshore wind farms’, Renewable Energy, vol. 224, p. 120184, Apr. 2024, doi: 10.1016/j.renene.2024.120184.
- M. A. Hannan, M. M. Hoque, A. Mohamed, and A. Ayob, ‘Review of energy storage systems for electric vehicle applications: Issues and challenges’, Renewable and Sustainable Energy Reviews, vol. 69, pp. 771–789, 2017.
- S. Tanuma, T. Shiratori, T. Kimura, K. Goto, S. Ichimura, and C. J. Powell, ‘Experimental determination of electron inelastic mean free paths in 13 elemental solids in the 50 to 5000 eV energy range by elastic‐peak electron spectroscopy’, Surface & Interface Analysis, vol. 37, no. 11, pp. 833–845, Nov. 2005, doi: 10.1002/sia.2102.
- Y. Yang, Q.-S. Jia, X. Guan, X. Zhang, Z. Qiu, and G. Deconinck, ‘Decentralized EV-based charging optimization with building integrated wind energy’, IEEE Transactions on Automation Science and Engineering, vol. 16, no. 3, pp. 1002–1017, 2018.
- J. Van Mierlo, P. Van den Bossche, and G. Maggetto, ‘Models of energy sources for EV and HEV: fuel cells, batteries, ultracapacitors, flywheels and engine-generators’, Journal of power sources, vol. 128, no. 1, pp. 76–89, 2004.
- Q. Wu et al., ‘High-performance all-polymer solar cells with only 0.47 eV energy loss’, Sci. China Chem., vol. 63, no. 10, pp. 1449–1460, Oct. 2020, doi: 10.1007/s11426-020-9785-7.